Multi class case
Contents
5.4. Multi class case#
5.4.1. Naive idea (one-vs-all)#
Assume that there are \(N\) classes. The naive idea is to decompose this \(N\)-class classification problem into \(N\) binary classification problems. The model will contains \(N\) classifiers. The \(i\)th classifer is used to classify whehter the given features has the label i
or not.
For example, asusme we are dealing with the iris
dataset. There are 3 classes. By the naive idea, we will modify the labels into Setosa
and not Setosa
, and use it to train the first classifier. Similarly we can have two more classifiers to tell Versicolour
/not Versicolour
and Virginica
/not Virginica
. Then we combine all three classifiers to get a final classifier to put the data into one of the three classes.
5.4.2. Softmax
function#
A better method is mimic the sigmoid function. Recall that in binary classification problem, after
the sigmoid function is applied \(p=\sigma(z)\). This \(p\) is interepreted as the probability for the data belonging to class \(1\). For \(N\)-class problem, we could generalize the sigmoid function to softmax
function, whose value is a \(N\)-dimensional vector \(p=[p_k]_{i=1}^N\). Here \(p_k\) represents the probability for the data belonging to class \(k\). Then after we get the vector \(p\), we then find the highest probability and that indicates the class of the data point.
The softmax
function is defined in the following way:
In the model, each \(z_i=L_i(x)=\theta^{(i)}_0+\sum_{i=1}^n\theta^{(i)}_ix_i,\) has its own weights.
The related cost function is also updated:
Therefore the same gradient descent algorithm can be applied.
Note
Note that sigmoid
function and the binary crossentropy
cost functions are the special case of softmax
function.
Note
The labels are not recorded as labels, but as vectors. This is called dummy variables, or one-hot encodings.
5.4.3. Codes#
Both
LogisticRegression()
andSGDClassifier()
by default uses the one-vs-all naive idea.Using
kears
,softmax
can be implemented. The key configuration is the loss functionloss='categorical_crossentropy'
and the activation functionsoftmax
. Note that in this case the labels should be translated into one-hot vectors.
We use make_classification
as an example. To save time we won’t carefully tune the hyperparameters here.
from sklearn.datasets import make_classification
from sklearn.model_selection import train_test_split
X, y = make_classification(n_samples=1000, n_features=10, n_informative=2, n_redundant=2, n_repeated=2, n_classes=3, n_clusters_per_class=1)
X_train, X_test, y_train, y_test = train_test_split(X, y, test_size=0.15)
Although there are totally 10 features, the dataset can be visualized using the informative features. By description, the informative features are the first two.
import matplotlib.pyplot as plt
plt.scatter(X[:, 0], X[:, 1], c=y)
<matplotlib.collections.PathCollection at 0x1d3eff72b90>
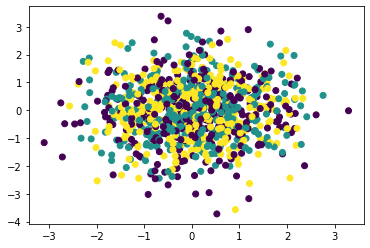
from sklearn.linear_model import LogisticRegression
clf = LogisticRegression()
clf.fit(X_train, y_train)
clf.score(X_test, y_test)
0.8733333333333333
from sklearn.linear_model import SGDClassifier
clf = SGDClassifier()
clf.fit(X_train, y_train)
clf.score(X_test, y_test)
0.8733333333333333
To apply keras
package, we should first change y
into one-hot vectors. Here we use the function provided by keras
.
from tensorflow.keras.utils import to_categorical
vy_train = to_categorical(y_train, num_classes=3)
vy_test = to_categorical(y_test, num_classes=3)
from tensorflow.keras import models, layers
model = models.Sequential()
model.add(layers.Dense(3, activation='softmax', input_dim=10))
model.compile(optimizer='adam', loss='categorical_crossentropy', metrics=['accuracy'])
model.fit(X_train, vy_train, epochs=50, batch_size=50, verbose=0)
_ = model.evaluate(X_test, vy_test)
1/5 [=====>........................] - ETA: 0s - loss: 0.4436 - accuracy: 0.9062
5/5 [==============================] - 0s 2ms/step - loss: 0.4380 - accuracy: 0.8733